Introduction - Fluorescence microscopy once relied on single plane images from relatively small areas and yielded limited amounts of quantitative data. Nowadays, many imaging experiments encompass some form of depth or a Z-stack of images, often from distinct regions in the sample. Hence, much like biochemical and molecular experimental datasets2, accurate normalization, beyond background subtraction3 of imaging signals, could reduce tissue-derived and/or technical variation. Specifically, 2-Photon (2P) and Light-Sheet (LS) microscopes enable the acquisition of images from both deep and wide tissue dimensions (Fig 1a, c, left panel). However, every biological sample and imaging technique introduces its own acquisition aberrations: beyond mirror and lens distortions4, the imaged preparations combine different characteristics (of e.g. cell density and lipid composition) that affect the optical penetration and light scattering at diverse tissue depths. Experimental limitation (antibody penetration, clearing efficiency) also constrain the ability to extract information from imaging experiments. Taken together, these difficulties call for the development of universal post-acquisition image correction/normalization tools that account for signal-carrying pixels and which estimate the specific heterogeneity of each image individually. To achieve 3D normalization, we developed a new algorithm, Intensify3D (Fig 1. b), Intensify3D aims to detect and use the background for correct normalization of the signal. Consequently, our normalization algorithm initiates with an estimation of the background by removing as much as possible of the imaged signals (as defined by the experimenter). Then, the background intensity gradients are used for correction by local transformation (correction by division) of both signal and background, without compromising the signal-to-noise ratio (Fig 1. a, b).
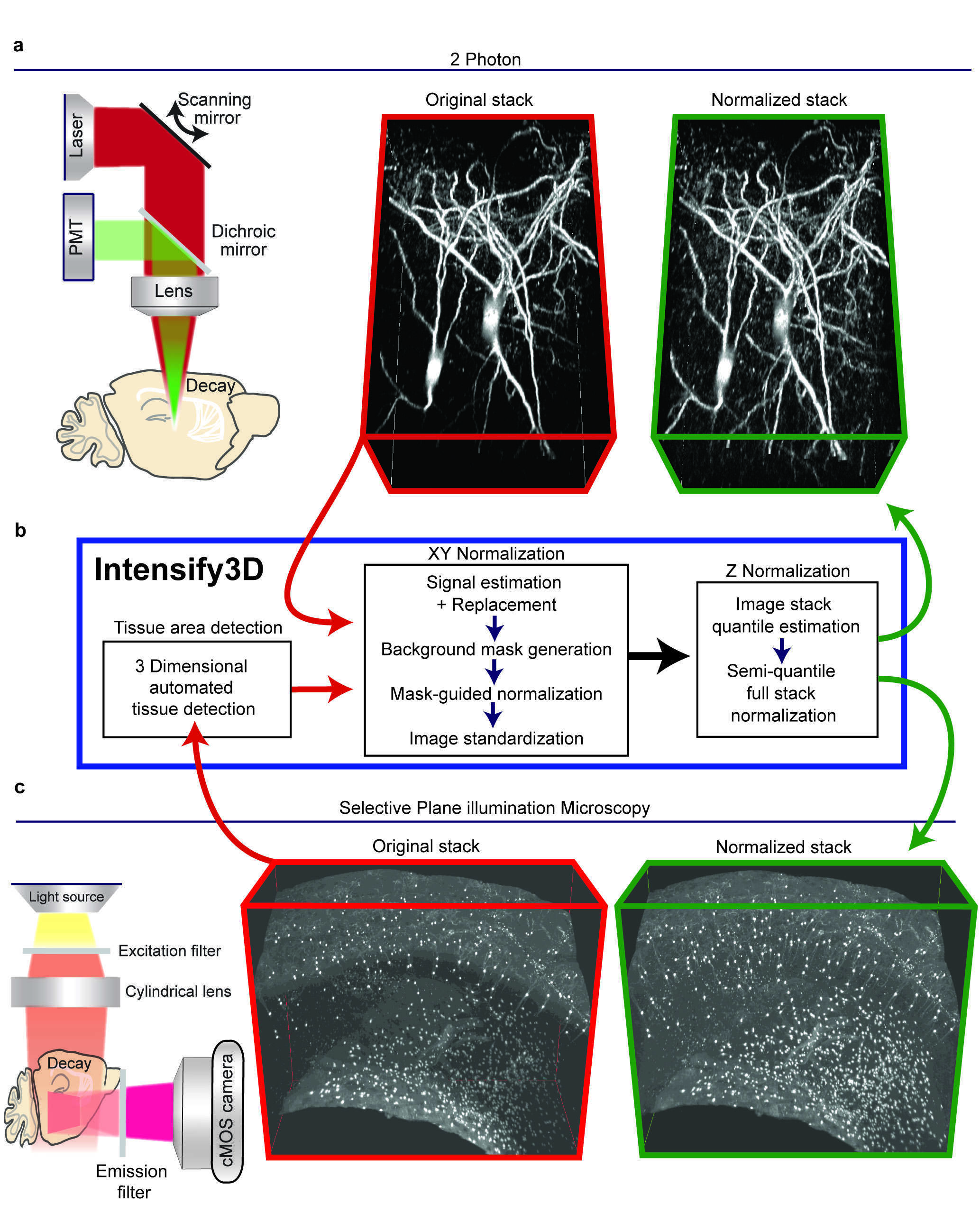
Figure 1. The basic normalization process of Intensify3D for 2-Photon and Light-Sheet 3D imaging. a. Left panel. 2-photon imaging setup illustrating the decay in excitation laser (red) and emitted light (green) through the imaged tissue. Red frame, middle panel. 3D projection of In vivo 2-photon Z-stack of cortical cholinergic interneurons (CChIs) up to 300µm depth, bottom portion is deeper. Green frame, right panel. 3D projection of image stack post normalization with Intensify3D; note the enhanced visibility of deep neurites. b. Intensify 3D processing pipeline for 2-Photon and light sheet image stacks. The latter requires an additional step to only account for tissue pixels in the image. The images in the stack are normalized one by one (XY normalization). After all the images are corrected the entire stack is corrected (Z Normalization) by semi-quantile normalization (other options exist). c. Left panel. Light-Sheet imaging setup where the excitation light is orthogonal to the imaged surface. Red frame, middle panel. iDISCO immunostaining and clearing of CChIs as well as striatal Cholinergic interneurons. Original image suffers from fluorescence decay at increasing tissue depth. Green frame, right panel. Intensify3D Normalized image stack. Images before and after normalization are presented at the same brightness and contrast. levels.
Results - We tested Intensify3D in two state-of-the-art imaging platforms (2-Photon and Light-Sheet) and demonstrated that it can overcome common sample heterogeneity in large image stacks using both of these technologies and correct significant dataset errors. Specific advantages of our algorithm include its capacity to distinguish between the signal and background with minimal parameters defined by the experimenter and avoiding distorting one at the expense of the other, as well as enabling applicability to various imaging platforms. The resulting avoidance of imaging errors and improvements in signal homogeneity are therefore an important asset for fluorescence microscopy imaging studies of all cells and tissues, especially in the brain. 2-photon imaging - Intensify3D normalization enabled homogenous representation across the entire image stack. Additionally, Intensify3D corrected significant errors in the estimation of deep dendrite diameters. Thus, normalized images offer a better representation of both imaged cell bodies and their thin extensions and serve as a superior platform for reconstructions and possibly modeling of the electrical properties of these neurons. Hence, this algorithm may offer a special added value to world-wide leading brain research projects. Light-Sheet imaging - Appling Intensify3D on LS data obtained from cleared5 adult mouse brains dramatically improved the detection and visualization of the barrel fields, indicating its applicability for such studies. At the microscale, we demonstrated that post-normalized scans of detected CChIs somata represent their real-life density, distribution, and composition compared to original scans, highlighting the importance of image normalization. Finally, to test the applicability of Intensify3D to diverse tissues we selected the mammary gland and heart, both of which present considerable challenges. We showed that with normalization we could extract the morphology of the milk ducts and buds by “simple” auto fluorescence.
Conclusions - Our current findings and analyses demonstrate that the Intensify3D tool may serve as a user-guided resource, correct sample- and technology-driven variations, improve the reproducibility, and add extractable information to numerous imaging studies in neuroscience research as well as in life sciences at large. Given these advantages, we hope that our work will open an active discussion on matters of image normalization. We believe that image normalization has an integral role in any imaging experiment where numerical data is extracted. As in other fields of life sciences, normalization reduces variability between samples even when the experimental conditions are superb. Finally, Intensify3D might further be of value to time lapse fluorescence imaging platforms such as time lapse structural imaging or calcium imaging in which the fluorescence of the imaged sample is often compromised during imaging.
- Yayon, N. et al. Intensify3D: Normalizing signal intensity in large heterogenic image stacks. Sci. Rep. 8, 4311 (2018).
- Bolstad, B. M., Irizarry, R. ., Astrand, M. & Speed, T. P. A comparison of normalization methods for high density oligonucleotide array data based on variance and bias. Bioinformatics 19, 185–193 (2003).
- Dunn, K. W., Kamocka, M. M. & McDonald, J. H. A practical guide to evaluating colocalization in biological microscopy. Am. J. Physiol. Cell Physiol. 300, C723-42 (2011).
- Dong, C.-Y., Koenig, K. & So, P. Characterizing point spread functions of two-photon fluorescence microscopy in turbid medium. J. Biomed. Opt. 8, 450–459 (2003).
- Renier, N. et al. iDISCO: A Simple, Rapid Method to Immunolabel Large Tissue Samples for Volume Imaging. Cell 159, 896–910 (2014).