PURPOSE: Reliable evaluation of fetal development is essential to reduce short and long-term risks to the fetus and the mother. MRI is increasingly used to provide accurate fetal structural and functional information. However, fetal MRI diagnosis is hampered by the need to manually extract morphological measures, which requires the segmentation of the fetal envelope. Manual delineation of complex structures, e.g., the fetal envelope is a tedious, error-prone, and time-consuming task. Automatic segmentation often requires expert corrections. Interactive methods are best suited for complex structures since they allow slice-by-slice segmentation validation and correction.
METHODS: We have developed a new method for the interactive segmentation of volumetric medical images based on real-time fine-tuning of a Fully Convolutional Network (FCN). Our method uses a FCN to perform the segmentation with a small number of training examples during user-driven interactive segmentation. It performs an initial segmentation on a slice, which is then manually corrected by the user. The resulting user-validated segmentation is then used to fine-tune in real time the FCN to improve the initial segmentation of the following slices.
To demonstrate our method on fetal body envelope segmentation, we collected 1,741 slices from 21 fetal MR scans of fetuses with 18-37 weeks` gestation with a 1.5T GE Signa Horizon scanner using a custom protocol. We performed three studies: 1) manual delineation of the fetal body by two expert radiologists to obtain the ground truth segmentations; 2) initial segmentation with the global network and manual correction with no global network fine-tuning; 3) same as 2, but with global network fine-tuning after the segmentation correction in each slice. Evaluation measures were the segmentation correction time and the segmentation accuracy -- Volume Overlap Difference (VOD).
RESULTS:
1) the mean manual delineation time was 68 mins (std=6); the mean inter-observer VOD variability was 9.5% (std=1.4). 2) the mean initial segmentation VOD was 34.8% (std=3.7); the mean segmentation corrections time 59.9 mins (std=5.5), a reduction of 8.1 mins (12%) wrt the manual delineation with the same VOD. 3) the mean segmentation correction time was 4.7mins (std=0.5), a mean reduction of 92.2% (std=2.4) with a mean final VOD of 10.3%. Each interactive step took an average of 1.2±0.2 secs, thereby enabling an interactive user experience.
CONCLUSION: Interactive segmentation using real-time fine-tuning of a FCN is a powerful tool that can be used to decrease segmentation time by up to x20 without compromising the quality of the segmentation.
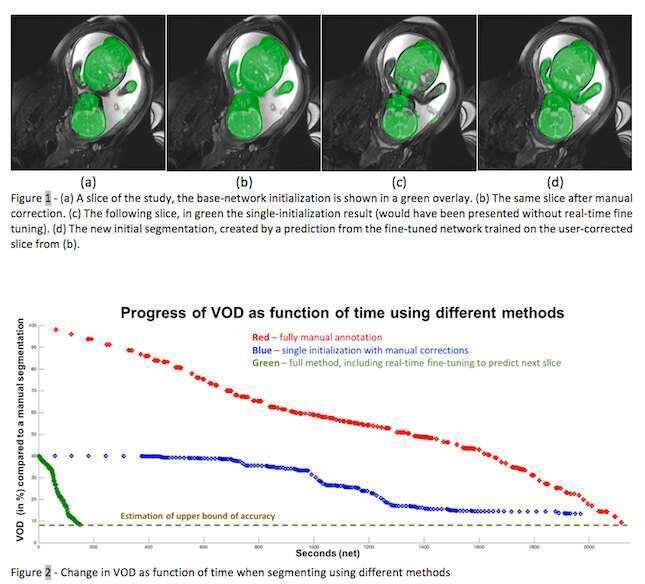